Architecture in the age of AI is one of four scans that forms part of the Technological Innovation theme for RIBA Horizons 2034.
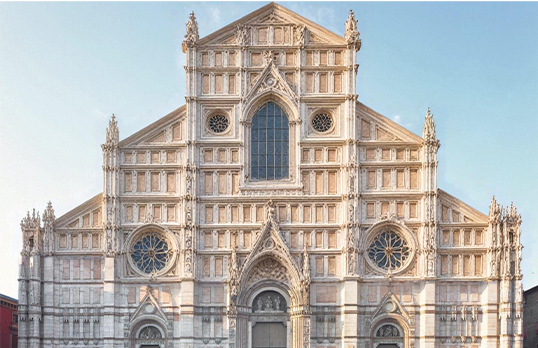
Let’s perform a thought experiment. Imagine that it is 2034, and artificial intelligence (AI) has turned out to be an enormously beneficial force in the practice of architecture.
Recently completed projects are noticeably safer, more harmonious and more sustainably constructed. The use of AI throughout architectural firms has enabled an explosion of design creativity, coupled with a more collegial relationship with engineers and builders. Even the smallest and most routine structures bear the touches of thoughtful design.
Clients are delighted, professional employment is stable, and fees exhibit steady growth commensurate with the greater overall value that the profession brings.
Architects and their staff routinely work collaboratively with a suite of AI-enabled software that allows them to access an immense bank of empirical and theoretical knowledge concerning how the built environment can support human practices and aspirations. They routinely leverage AI-based capabilities to fluidly support all phases of interaction with clients and builders in building delivery.
This vision is the ‘Ideal Outcome’ for AI and architecture in 2034. To have reached this goal, what issues must have been solved along the way? What signs would indicate that progress was being made?
To answer these questions requires a basic understanding of how modern AI works.
How AI works
AI has historically been concerned with giving computers sufficient knowledge of relevant aspects of the world to perform tasks that require some degree of intelligence.
Until the early 2000s, the dominant AI method was to use complex and obscure mathematical tools to manually author thousands of distinct statements about the world, download these statements into computers, and then employ specialised AI software to process and combine these statements to support intelligent behaviour.
This method was laborious and difficult to scale, had severe challenges encoding uncertain or imprecise knowledge, and typically resulted in systems with narrow and often very brittle capabilities.
The great revolution in AI over the past 20 years has been the development of machine learning algorithms. These take advantage of immense amounts of computational power to process internet-scale quantities of text and imagery, automatically derive knowledge of the world from correlations in this data set, and encode this knowledge into enormous and opaque numerical structures.
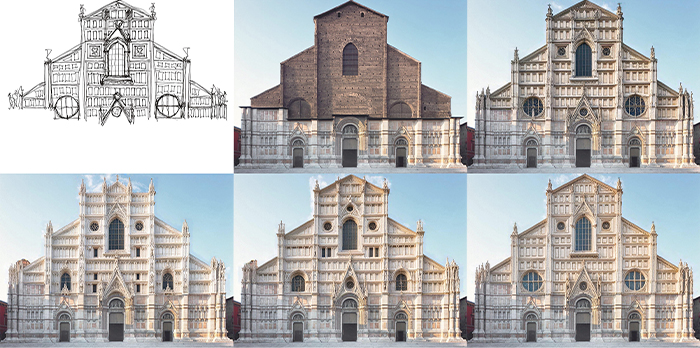
These algorithms have been astonishingly effective. [1] Using these techniques, computers have quickly become remarkably fluent in language and reasoning and have acquired subtle information about human experience from deep patterns in human communication.
Current AI systems – those trained using machine learning – leverage these patterns in ways that are not fully understood. They enable computers to exhibit human-like conversational behaviour, demonstrate superhuman skills across many tasks, acquire new capabilities, create novel artefacts, and make new scientific discoveries.
Four signposts
Given this capability, how will the worlds of architecture and AI intersect in 2034, and what early signs of change will indicate progress towards the Ideal Outcome?
Scientists have observed that machine learning systems appear to follow ‘scaling laws’ that quantify how capability might increase in AI systems as they learn from more and more data. [2] These scaling laws allow AI companies to predict how much capital and computing power they need for a particular level of desired capability.
For our purposes, these scaling laws undergird a set of four fundamental signposts that allow us to monitor progress towards AI capabilities that would enable the Ideal Outcome.
1. Acquiring professional knowledge
The first of these signposts involves the acquisition by AI systems of characteristically architectural data and epistemology.
In The Future of the Professions, Richard and Daniel Susskind argue that “knowledge asymmetry” between professionals and their clients and the hoarding of “practical knowledge” is fundamental to professionals maintaining their status as experts. They also argue that computation and the internet have the potential to redress the imbalance between the providers of professional judgement and their consumers. [3]
Modern AI has this potential. The AI scaling laws show that increasing AI capability depends strongly on its ability to train on increasing quantities of relevant text, imagery and other data. In their ceaseless quest for data on which to train their AI systems, AI companies have already mined huge swaths of text, images, and video from the internet, and have leveraged the contents of the world’s great libraries and information repositories.
AI systems will soon have absorbed most of the world’s publicly accessible essays, textbooks, curricula, blog posts, and media concerning architecture and related topics, and will have derived an immense amount of knowledge of what works and what doesn’t.
This has given rise to ferocious battles about the degree to which the use of this information for AI training falls under the doctrine of fair use and fair dealing. AI’s dependence on vast amounts of training data certainly risks transferring resources from those who create to companies who use their creations to train models that supplement or replace the creators. The legal status of training AI systems from copyrighted architectural designs or buildings is not currently settled. [4]
However, in the field of architecture, much of the Susskinds’ “practical knowledge” is locked away in firms’ private repositories of artefacts such as contracts, sketches, correspondence, standards, floor plans, building sections, and 3D representations (such as digital models, renderings, and analytical and physical models).
Furthermore, these artefacts are based on a specialised epistemology and set of abstractions, in which junior architects gain fluency through the human process of architectural education and workplace mentoring.
For AI systems to acquire this critical practical knowledge of the profession, and thereby gain capability in the processes needed to operate in a firm, this private knowledge must be made available for AI systems to train on.
In domains like medicine and law, we are already starting to see commercial AI systems that claim to safely combine the power of general-purpose AI models with an individual business’s proprietary data. An important signpost for this capability would be seeing powerful AI systems being marketed to architectural practices which can incorporate firm-specific proprietary data and artefacts.
2. Achieving human-like judgement
A second signpost for progress towards the Ideal Outcome involves AI systems achieving the capability to interact professionally and to form professional judgements from uncertain information.
Architects rely daily on a refined intuition to guide subtle decisions about imprecise tradeoffs and engineering constraints, and to account for ill-defined ethical, cultural, and perceptional interpretations. Not only do they need the skill to make design decisions, but they must also persuasively defend their decisions to a client who will choose, experience, and pay for it.
Today’s AI systems have rudimentary capabilities in these areas, but they are not yet close to acquiring the insight and awareness of the human context that is characteristic of an experienced human architect.
Still, AI systems are on a trajectory to develop a level of proficiency in areas like these. AI-enabled assistants for scientists, lawyers, and other professionals are starting to emerge commercially, as are early (and somewhat creepy) AI-based social and romantic partners that exhibit early signs of emotional intelligence.
More fundamentally, many of the largest AI companies are actively working on how to modify the behaviour of their systems to match enumerated human values. This is so that their systems exhibit behaviour consistent with standards of fairness, cooperation, truthfulness, and the like. This type of work is referred to in the AI industry as “alignment”. [5] Progress here would be a clear indicator that the Ideal Outcome is becoming more possible.
3. Integrating into business
A third signpost for progress towards the Ideal Outcome involves agreement on how to best integrate AI systems business contexts.
AI systems can greatly speed up tasks and allow humans to be more efficient. Examples of professional tasks that AI can perform in seconds with an impressive degree of quality include:
- creating initial good drafts of essays and documents
- generating images and video renderings
- answering questions
- following chains of reasoning
- checking artefacts for compliance and other properties
- summarising large amounts of material
- exploring the implications of specific design choices
AI image generators lack spatial understanding but are beginning to show partial spatial awareness, as demonstrated by the below images of a living space, into which the designer was asked to insert mirrors.
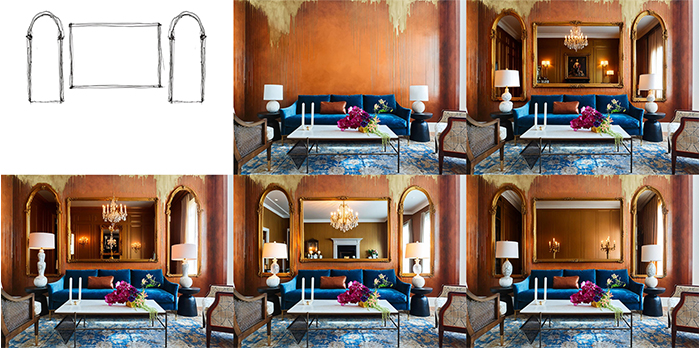
As AI-based computation displaces the human intellectual effort to perform architecturally relevant tasks, the cost of providing architectural services could be substantially reduced, possibly leading to a corresponding reduction in firm staffing and the fees architects can charge. Significant advances in automation technologies have often dramatically displaced workers (the Luddite era is a well-known example), especially during the transition period.
But some argue that this is too superficial. The scholar Kathryn Lofton, for example, has cautioned against the “delusion of efficiency,” and reminds us that the introduction of powerful new technologies often results mostly in a rebalancing of job requirements and stimulates the introduction of new jobs that are required to take maximum advantage of the new technology.
In short, it is currently unclear how the integration of AI into architectural practice will impact staffing and fees. AI is just starting to have a real involvement in different sectors of the economy. There is very little data that would allow us to confidently predict how the rhythms of architectural work will evolve in the face of powerful new AI systems.
One signpost to watch, though, is the rate of introduction of new AI-specific jobs, especially in architecture. The advent of AI systems has already produced several new job categories (such as ‘prompt engineer’ and ‘AI auditor’) as businesses experiment with ways to integrate AI systems. This suggests that using AI in the context of an architectural firm will bring with it a reordering of jobs instead of simply displacing architects.
4. Clarifying professional responsibility
A fourth signpost for progress towards the Ideal Outcome involves clarifying the relationship between professional responsibility and intelligent machines.
A fundamental characteristic of professionalism is personal, human responsibility – being on the hook. Critical concepts of obligation, culpability, duty, and trust are currently rooted in a social contract where the morally accountable actors are humans acting in specific roles. AI systems can exhibit superhuman knowledge and abilities, but they are currently treated exclusively as helpers to humans, and humans still have ultimate accountability for actions.
However, the Susskinds foresee a “post-professional society” where knowledge and expertise reside not just in people, but also in machines. [6] In such a society, it is vital to be explicit about where responsibility lies. The Grenfell disaster in London was attributed to the dissolution of professional responsibility in the complex web of decisions, material choices, and failures of the building delivery chain, simultaneously making everyone and no one responsible.
Therefore, a signpost for progress towards the Ideal Outcome is a more careful delineation of responsibility between humans and highly capable AI software. If we see examples of actual delegation of legal accountability to a piece of software (instead of always to a person who serves as a professional guarantor of its outputs), this would be an indicator of the Ideal Outcome.
AI, architecture, and society
The dramatic development of AI over the last decade suggests that a transformational impact on architectural practice is on the horizon. However, the signposts we propose demand that architects consider issues beyond raw AI capability on the way to achieving the Ideal Outcome.
The pace of AI development currently exceeds the ability of wider society to absorb and come to a consensus on those developments. Although the computational capability needed to achieve these signposts might be available by 2034, each of the signposts also involves deep collective tradeoffs, which will be resolved in different ways and at different speeds in different societies.
Furthermore, the impact of AI on architectural practice will not develop in a vacuum – it will have a tremendous impact on the overall ways that we live and work, and this will inevitably shift our goals for the built environment. How will the design of physical spaces need to change to reflect this changing nature of human work and leisure? [7] How will possibilities for these spaces evolve as it becomes feasible to embed AI and robotics into the building itself?
The ways we create, build, use, and experience space in an AI-enabled world, as well as how the built environment's purpose will transform, are difficult to anticipate. They will play out over decades as the dividing line between physical and digital experience continues to blur in the information age.
AI capabilities will be enormously advanced by 2034. Their impact will drive changes in architectural practice and coordinating these changes will be the joint work of all the players in the architectural ecosystem.
Working to realise the Ideal Outcome will become increasingly critical as the architectural profession strives to tackle the larger challenges addressed in the RIBA Horizons 2034 scans, including creating a built environment fit for a changing population amid a climate emergency. The signposts proposed here will help the profession monitor and evaluate the growing impact of AI over the next 10 years.
Author biography
Mark Greaves is currently Executive Director of the AI and Advanced Computing Institute at Schmidt Sciences. Before that, he was a senior leader in AI and data analytics within the National Security Directorate at Pacific Northwest National Laboratory, where he created and managed large research programs in AI on behalf of the US government.
Previously, Mark was Director of Knowledge Systems at Vulcan Inc., Director of DARPA’s Joint Logistics Technology Office, and Program Manager in DARPA’s Information Exploitation Office. He has published two books and over 40 papers, holds two patents, and has a PhD from Stanford University.
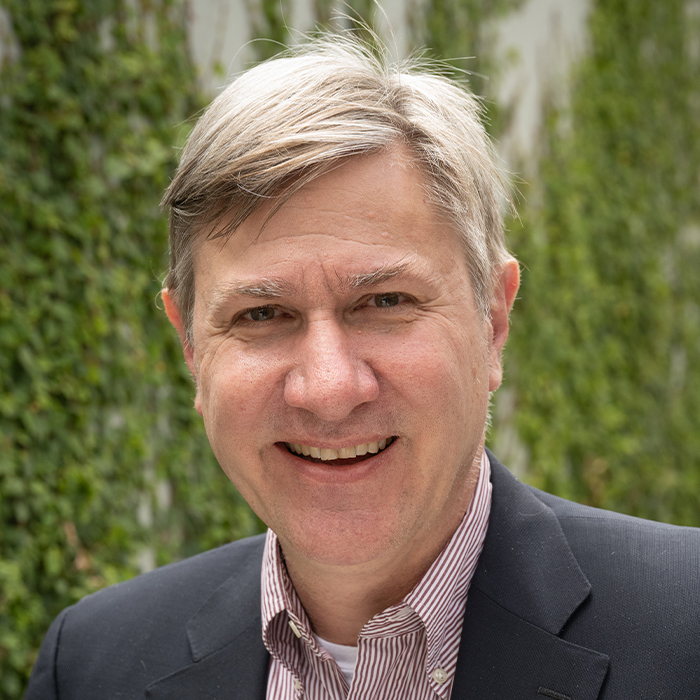
RIBA Horizons 2034 sponsored by Autodesk

References
[1] A. Halevey, P. Norvig and F. Pereira (2009). The Unreasonable Effectiveness of Data, IEEE Intelligent Systems 24:2
[2] J. Kaplanet et al. (2020). Scaling Laws for Neural Language Models
[3] R. Susskind and D. Susskind (2022). The Future of the Professions: How Technology Will Transform the Work of Human Experts, Updated Edition, 16–24. Oxford University Press
[4] The New York Times - N.N. Grynbaum and R. Mac (27 December 2023). The Times Sues OpenAI and Microsoft Over A.I. Use of Copyrighted Work
[5] OpenAI (n.d.). Superalignment
[6] R. Susskind and D. Susskind (2022). The Future of the Professions: How Technology Will Transform the Work of Human Experts, Updated Edition, 15. Oxford University Press
[7] P. Bernstein, M. Greaves, S. McConnell, and C. Pearson (2022). “Harnessing Artificial Intelligence to Design Healthy, Sustainable, and Equitable Places.” Computer 55:2, 67–71